Frank van Harmelen is professor of Artificial Intelligence at VU University Amsterdam and sits on the advisory committee for the Ammodo Science Awards. Since 2020, he has been director of the Hybrid Intelligence Centre, which researches AI systems for the future. 'Hybrid intelligence is about successful cooperation between humans and machines, where the whole is more than the sum of the parts.'
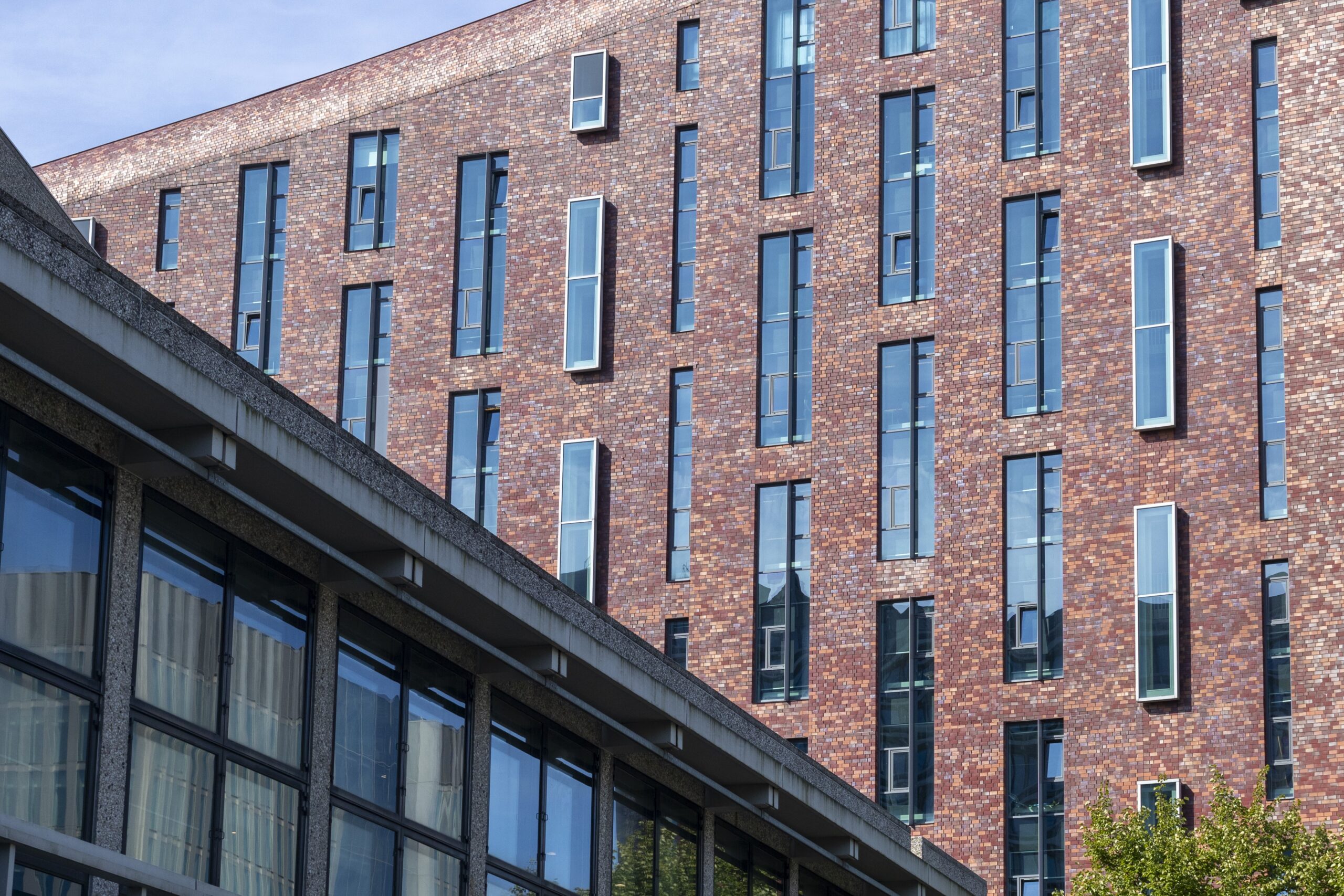
How would you describe the rise of AI?
For me, Artificial Intelligence (AI) is the most exciting field in computer science. Its history can be described as a kind of rollercoaster ride of great expectations and ambitions that resulted in both failures and wonderful discoveries. Some developments went faster than expected, others much slower. In the 1960s, for instance, American researchers confidently predicted that a computer could beat the world chess champion within five years. It finally succeeded only in 1997, almost 40 years later. But that was not yet the AI as we know it today.
What were the key developments?
In the 1980s, research mainly focused on the development of expert systems to support human specialists, such as doctors in making diagnoses. These models were manually programmed with thousands of decision rules, often with direct input from experts. Today, we work on a much larger scale and have systems with hundreds of millions of rules and huge medical databases, based on which AI can provide advice. At the same time, machine learning broke through in recent decades. In this, you don't input rules but AI learns to discover patterns on its own. Then you give the computer large amounts of examples, for example patient records, and then it learns for itself which drugs work for which diseases. So today there are two schools of AI: the knowledge-based approach, with millions of explicit rules, and machine learning, where computers learn from examples without instructions. The challenge now lies in merging these two approaches, which is complicated in practice by the differences in the underlying mathematics and methodology.
How did machine learning take off like this over the past decade?
This was possible because of two crucial factors: the availability of immense computing power and access to large data sets. In particular, large data sets are essential because machine learning depends on a plethora of training examples. For example, translating from English to Chinese cannot be fully described by rules, but if you have enough English texts and corresponding Chinese translations, the system can recognise patterns on its own. The past decade has also seen the emergence of deep learning, which allows computers to discover complex patterns in data that were previously invisible. This has spawned new applications such as facial recognition, machine translation and self-driving cars.
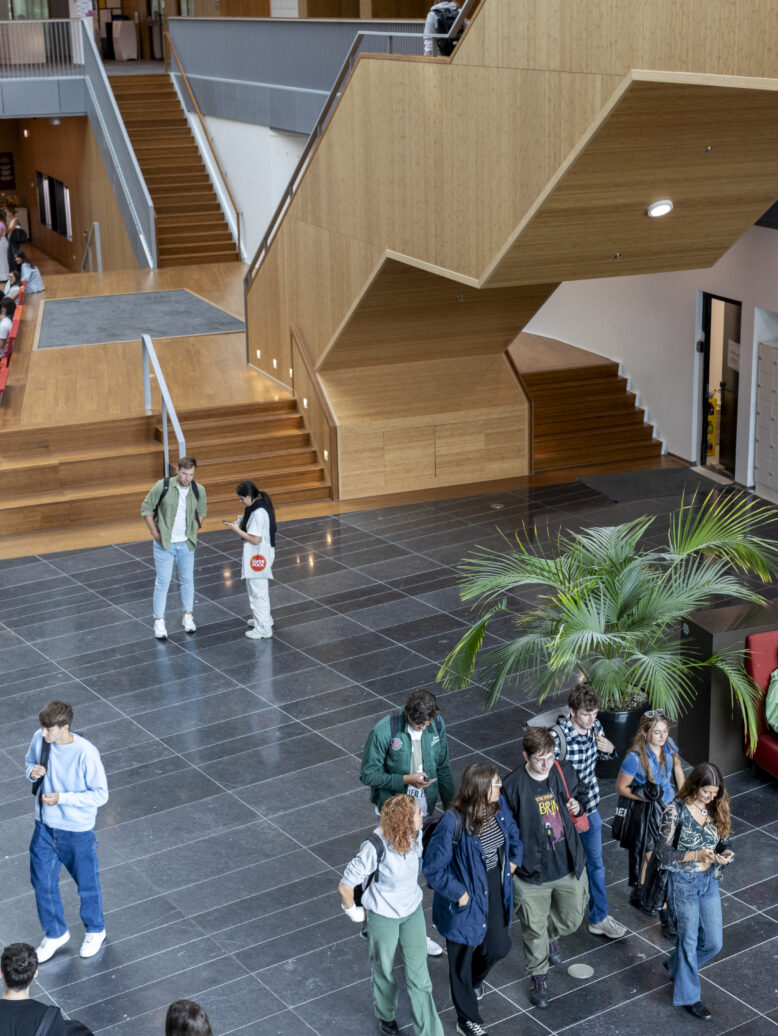
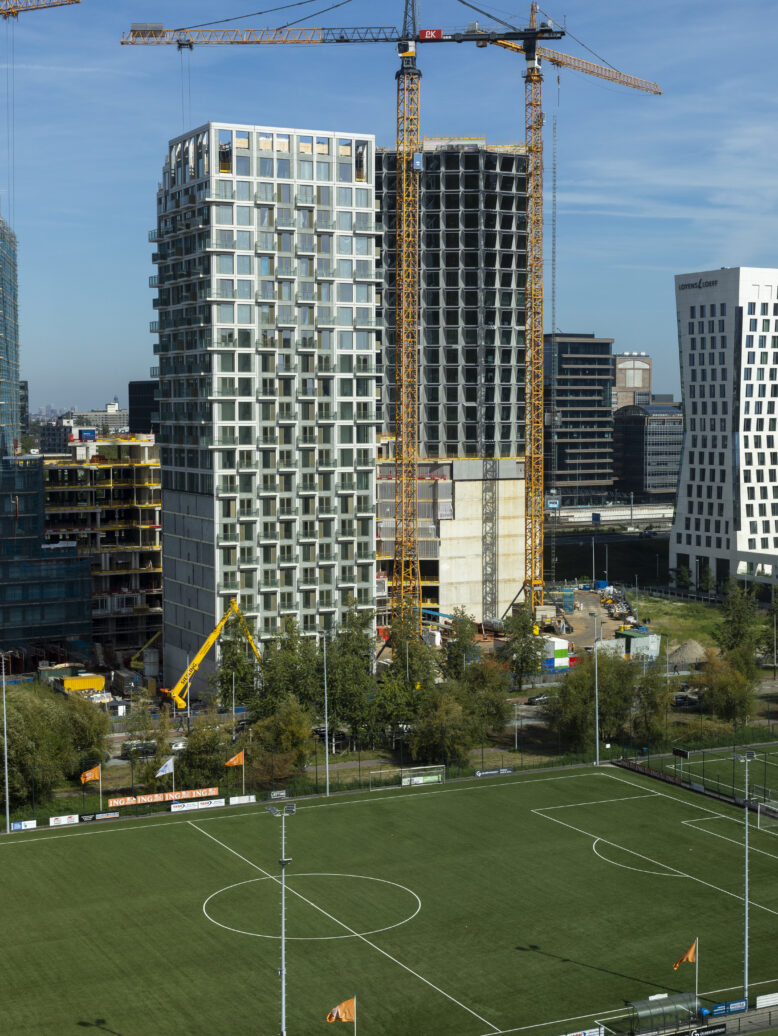
You are director of the Hybrid Intelligence Centre. What was the driving force behind the creation of this centre?
We launched in 2020 thanks to a €20 million Gravity Grant. Our goal is to develop AI systems that can work effectively with humans, resulting in hybrid teams where human and computer intelligence complement each other. Seven universities work together within the Hybrid Intelligence Centre, each with three or four research groups with their own areas of expertise. In total, we now have around eighty PhD students so this gives us real clout. In 2020, we were pioneers with such large-scale and interdisciplinary research into hybrid intelligence. Good ideas are often developed at the same time, and now work on this topic is going on worldwide under different names: in Germany they call it Human Centric AI, and there is even a Stanford Centre for Human-Centered AI. There is a growing realisation that the future of AI is not about replacing humans, but about supporting them, making them more efficient and working at a higher level.
How is hybrid intelligence different from classical artificial intelligence?
The traditional approach to artificial intelligence focuses mainly on automation, i.e. taking over human tasks. But the idea that machine intelligence will eventually equal human intelligence is now outdated. Human and artificial intelligence differ considerably and are not easily interchangeable. Radiologists, for example, will not soon be replaced by computers, but in the future they will have to work with AI systems that make suggestions to improve their diagnostics. So instead of worrying about jobs being taken over by AI, we should explore how the two forms of intelligence can complement each other. Computers have perfect memory, are impartial and can recognise patterns in large data sets. Humans, on the other hand, can show empathy and understand subtle contextual information. Hybrid intelligence is all about the collaboration between humans and machines, where the whole is more than the sum of the parts.
Does this change our definition of what intelligence is?
Intelligence is a concept that is ever-changing and that we are getting to grips with. In computing, the original definition of intelligence was based on the Turing test, where a computer was considered intelligent if it was indistinguishable from a human in an imitation game. Today, we find the idea that human intelligence serves as the benchmark obsolete. Indeed, I now also consider the idea that we need a definition before we can talk about intelligence obsolete. Just as biology has no single definition of 'life', there is no single definition of intelligence within AI either. Therein lies the very strength, because as Einstein put it, 'If we knew what we were doing, it wouldn't be called research.' Humans and computers possess different forms of intelligence, and that is fine. Definition is the goal of the journey, not the beginning.
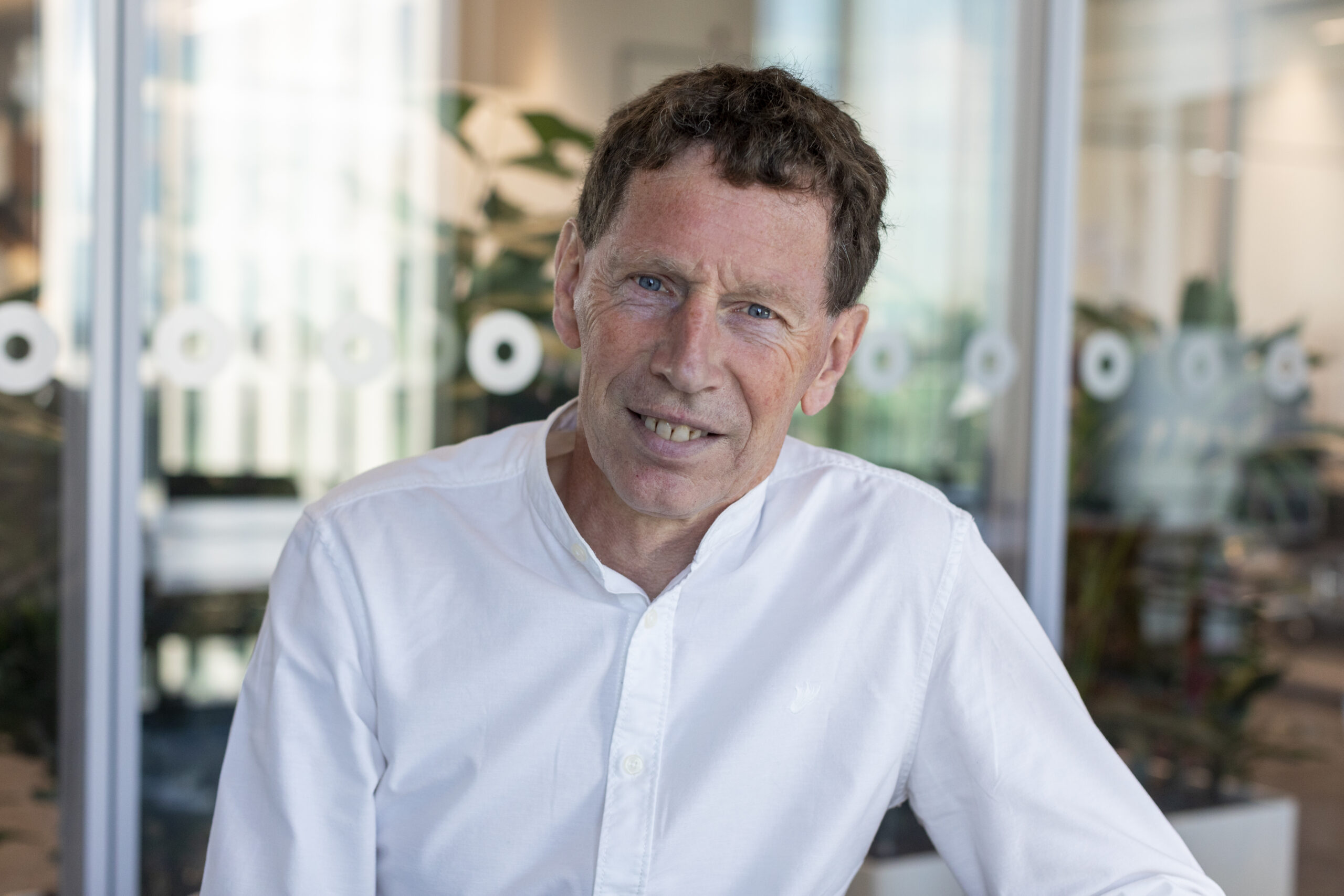
What will it take to make humans and AI work better together?
First of all, we need to ensure that both forms of intelligence understand each other well. This is still quite difficult, as machine learning often leads to a 'black box' where it is unclear how the system arrives at an answer. An example is AlphaGo, an AI model that won the board game Go by making moves that were initially incomprehensible, but 50 moves later turned out to be crucial. When you ask the computer why it made a particular move, you do not receive a neatly worded answer, but an incomprehensible list of number. At the moment, AI is not yet able to make that internal process understandable to us. That is why intensive work is currently being done to develop 'explainable AI', with the aim of making AI more understandable to humans.
And vice versa: what could AI learn from humans?
People have countless unspoken rules among themselves, such as the way we hold conversations: we tune into our interlocutor, let the other person finish talking, and so on. This 'common sense knowledge' is self-evident to us, but is far from logical to a computer. This is known as Moravec's paradox: things that are intuitive for humans are challenging for computers, and vice versa. This seemingly simple human behaviour serves as a lubricant in social interactions and is an essential element in teamwork. Therefore, truly successful human-computer cooperation becomes possible only when computers can also adhere to these social conventions.
How can an AI model learn that human behaviour?
That is a challenge. It includes the use of machine learning, in which computers analyse thousands of novels to recognise human behaviour patterns. After all, these describe all kinds of human behaviour, from love to conflict to irrationality. This process is similar to how children learn the meanings of behaviour through repetition. These meanings are also highly culture-dependent, so an AI model based on Chinese literature will differ from one based on Western literature. Moreover, human behaviour is context-dependent, so there is no universal solution. Our goal is to make computers aware of their context so that they can adjust their behaviour themselves, just like humans do.
Have successful hybrid partnerships already emerged from it?
Sure, we have researchers experimenting with robotics in education, for example, where small robots in classrooms support children in their learning. These robots have endless patience and take over repetitive tasks, such as helping a child learn tables, giving teachers more time for individual attention. Another interesting project focuses on using hybrid intelligence in microsurgery. Here, digital cameras assist in extremely delicate operations, such as repairing small nerves. These cameras can already respond to spoken commands, such as 'now turn 10 degrees to the right,' allowing the surgeon to work very precisely. The goal in the future is to achieve hybrid collaboration, where the camera is a full member of the surgical team and automatically understands what the surgeon needs, such as zooming in and out at the right times. This means the technology adapts to the human rather than the other way around.

Recently, ChatGPT has received a lot of attention. What made the launch possible?
The launch of ChatGPT in November 2022 dramatically changed our research field. The algorithm for training this kind of AI model, which applies machine learning to large amounts of text, had long been public and well-known. Thanks to huge investments in data, training and computing power, and with the support of companies like Microsoft, OpenAI, the company behind ChatGPT, has taken the technology to its current level. What followed from that massive scale-up is referred to within the AI community as 'emergent behaviour': unpredictable behaviour that is not consciously programmed. Even the creators still don't understand everything, as they train the model for A and it turns out to be suitable for B as well, without knowing beforehand exactly what B entails. This highlights the 'black box' nature of AI, where there is no clear explanation for the system's behaviour.
How is ChatGPT trained?
A language model like ChatGPT teaches something seemingly simple: the correct placement of words in sentences depending on the context. To do so, it uses a probability distribution for potentially matching words. For example, suppose the sentence starts with 'The farmer lives on the...'. Several words may follow at that point, such as 'yard', 'land' and so on. In this example, 'yard' has a higher chance of being selected because it has a strong association with 'farmer', while, for example, the word 'flat' has a weak association and thus has a lower chance of being selected. By making predictions about which words can be placed in a sentence in this way, ChatGPT generates responses. Interestingly, the model does not always choose the word with the highest probability value, otherwise it would always generate the same text. Instead, it throws a virtual die to randomly choose different but still reasonably likely words each time, giving you different answers to the same question.
What new risks are associated with these developments?
This powerful generative AI has many useful applications, but also unpredictable consequences. In particular, the phenomenon of hallucination, where the model generates misleading information, is a major risk. ChatGPT is built to always provide answers, often those answers are plausible but sometimes they are incorrect or even nonsensical. In situations where accurate information is vital, such as medical queries, ChatGPT's ability to provide false information can pose serious risks. The lack of warning about this is worrying, given the wide use of this technology. Another risk concerns ethical issues, such as the inadvertent surfacing of human biases in algorithms. The model is only as good as the data it is trained on. So if the data contains discriminatory elements, these may be reflected in ChatGPT's output, contributing to reinforcing stereotypes and discrimination. This is a serious problem that we as researchers need to be constantly aware of. Finally, there is the lack of explainability, which I mentioned earlier: we do not yet understand why the model knows some things and not others. The rapid implementation of this new technology, despite the risks present, means that we urgently need to start thinking about its responsible use.
How do we ensure responsible development of AI?
There are currently many conferences on FAT AI (Fair Accountable Transparent AI) discussing the agency of AI. ChatGPT so far only responds to questions, with no initiative of its own. Experiments with AI variants that do exhibit agency raise the question of whether regulation is needed for responsible development. Similar regulations already exist in other fields of science, such as biology with restrictions on human cloning and genetic modification of food. Within the Hybrid Intelligence Centre, we are also addressing this. We collaborate with another Gravity consortium called Ethics of Socially Disruptive Technologies (ESDIT), which focuses on ethical aspects of disruptive technologies such as robots and AI. Together, we mentor PhD students developing ethical guidelines for Explainable AI.
What developments do you expect in the coming years?
I stopped predicting the future in November, mainly because I could not have foreseen then that ChatGPT would appear in December. The AI field is developing at lightning speed. More progress is now taking place in a month than normally takes place in a year.
You are on the advisory committee for the Ammodo Science Award. How did you experience the selection process for the Ammodo Science Award for fundamental research 2023?
It was instructive and inspiring to see what standards of quality scientists from other fields apply. It forced me to rethink what I myself consider good science. Moreover, it was a lesson in humility, as all the candidates had done impressive work early in their research careers. Selecting two laureates from such a talented group was a challenge. In November, the advisory committees will meet again to discuss the nominations for the Ammodo Science Award for groundbreaking research 2024. I am already looking forward to the selection process where special attention will be paid to the power of collaboration within science.
Published on 19 September 2023.
Photos: Florian Braakman